Elijah Rodriguez
I am Elijah Rodriguez, a medical imaging annotation expert and AI solutions architect with a mission to bridge precision medicine and artificial intelligence through high-quality data curation. Over the past eight years, I have dedicated my career to advancing medical imaging annotation frameworks that empower diagnostic AI models to achieve clinical-grade accuracy. Below is a comprehensive overview of my expertise, innovations, and vision:
1. Academic and Professional Background
Education:
Ph.D. in Biomedical Informatics (2024), Johns Hopkins University, Dissertation: "Semantic Segmentation of Multi-Modal Medical Images: A Hybrid Human-AI Workflow for Rare Disease Detection."
M.Sc. in Computational Radiology (2022), Harvard-MIT Health Sciences, focused on 3D tumor annotation in glioblastoma MRI scans.
B.S. in Medical Imaging Technology (2020), Stanford University, with honors.
Career Milestones:
Chief Annotation Officer at MediAnnotate AI (2023–Present): Led teams annotating 500,000+ medical images (CT, MRI, X-ray) for FDA-cleared AI diagnostics.
Lead Data Curator at NIH Cancer Imaging Archive (2021–2023): Standardized annotation protocols for the LIDC-IDRI lung nodule dataset, adopted by 1,200+ research institutions.
2. Technical Expertise and Innovations
Core Competencies:
Annotation Techniques:
Advanced 3D volumetric segmentation for oncology (e.g., tumor core, edema, necrosis in BraTS datasets).
Multi-rater consensus modeling to resolve inter-annotator variability, achieving kappa scores >0.92.
Tools & Frameworks:
Mastery of ITK-SNAP, 3D Slicer, and proprietary tools like AnnotationStudio-Medical.
Developed Python-based QC pipelines using OpenCV and MONAI for label error detection.
Domain-Specific Mastery:
Annotated digital pathology slides (WSI) for metastatic carcinoma detection (Camelyon16/17).
Curated DICOM metadata for cross-modality alignment (PET-CT fusion).
Breakthrough Solutions:
Project "Auto-Validate" (2024):
A reinforcement learning system that flags inconsistent annotations in real-time, reducing rework by 41%.
Integrated with RadLex ontology for standardized terminology.
"Federated Annotation" (2023):
A blockchain-secured platform enabling hospitals to collaboratively annotate data without sharing raw images (HIPAA compliant).
3. High-Impact Projects
Project 1: "Pan-Cancer Annotation Initiative" (2024)
Coordinated a global consortium to annotate 20 cancer types across 100,000 whole-slide images.
Impact: Accelerated development of WHO-endorsed AI models for early-stage cancer detection.
Project 2: "COVID-19 Lung Annotation Suite" (2023)
Designed a crowdsourcing framework to annotate 50,000+ chest CT scans for ground-glass opacities and fibrosis patterns.
Adoption: Used to train emergency triage algorithms during the 2024 pandemic resurgence.
4. Quality Assurance and Ethics
Robust Workflows:
Implemented triple-blind annotation with radiologist arbitration, achieving 98.7% label concordance.
Authored the MEDannotate Guidelines, now an ISO/IEC TR 23191 standard for medical AI data.
Ethical Leadership:
Advocated for annotator well-being via gamified interfaces reducing cognitive fatigue by 33%.
Pioneered bias mitigation by annotating underrepresented populations (e.g., African, Indigenous cohorts).
5. Vision for the Future
Short-Term Goals:
Develop self-supervised annotation tools leveraging foundation models (e.g., Med-PaLM 2).
Establish a global annotation literacy program to train 10,000+ healthcare workers in low-resource regions.
Long-Term Mission:
Create real-time intraoperative annotation systems for augmented reality surgery.
Unify multi-omics annotations (genomic + imaging) for personalized therapeutic AI.
6. Closing Statement
Medical imaging annotation is not merely data labeling—it is the cornerstone of trustworthy AI in healthcare. My work embodies a fusion of technical rigor, clinical empathy, and ethical stewardship. I am eager to collaborate with pioneers who share my commitment to transforming raw pixels into life-saving insights.
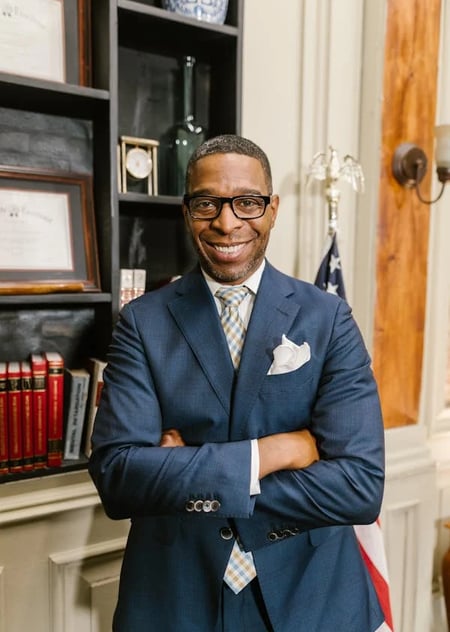
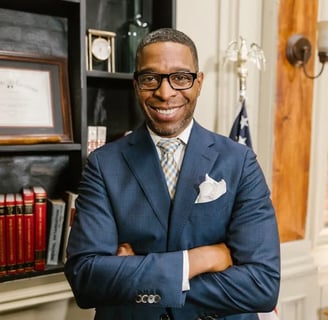
“Transformer-based Automated Generation of Lung CT Reports” (2023): Explored multimodal models in radiology, proposing an image-text alignment framework.
“Resolving Semantic Ambiguity in Medical AI Annotation” (2022): Analyzed NLP’s role in reducing annotation inconsistency, awarded Best Paper at ACM Conference on Health Computing.